Understanding the Clear Bounds for Data Products in the Organizational Data Mesh Journey | Issue #39
Demystifying the Blurry Boundaries of Data Products
Ayush Sharma is a Technical Lead and Sr. Consultant at ThoughtWorks, and we highly appreciate his contribution and readiness to share his knowledge among MD101 readers.
We actively collaborate with data experts and practitioners to bring the best resources to the community. If you have something to say on Modern Data practices and innovations, feel free to reach out to us! Note: All submissions are vetted for quality and relevance.
As the concept of data products gains momentum as a solution that helps organizations design scalable and flexible data architectures, the challenges of getting them right also come into greater focus. Data and engineering teams increasingly need to focus on data service-level agreements (SLAs) and create multiple data products that cater to different consumer needs.
This blog post draws on our experience of outlining challenges you are likely to face when defining the boundaries of data products. We dive deep into building concise and use case-focused data products, rather than creating a monolithic data set, to achieve greater agility, efficiency, and value delivery.
Before we get into that, though, I want to take a moment to establish two fundamental principles that will help address challenges more effectively:
First, a data product is the fundamental unit of the data mesh architecture, to be designed, built, tested, and maintained independently. Data products are:
Small, autonomous, and easy to understand, manage, and consume
Organized around business domains rather than technical concerns
Structured around a clear scope and well-defined set of responsibilities
In other words, the smallest feasible data product should provide value on its own, and its usage should be self-contained within that product. It should be owned and managed by the domain teams with the domain knowledge and expertise to create high-quality data products.
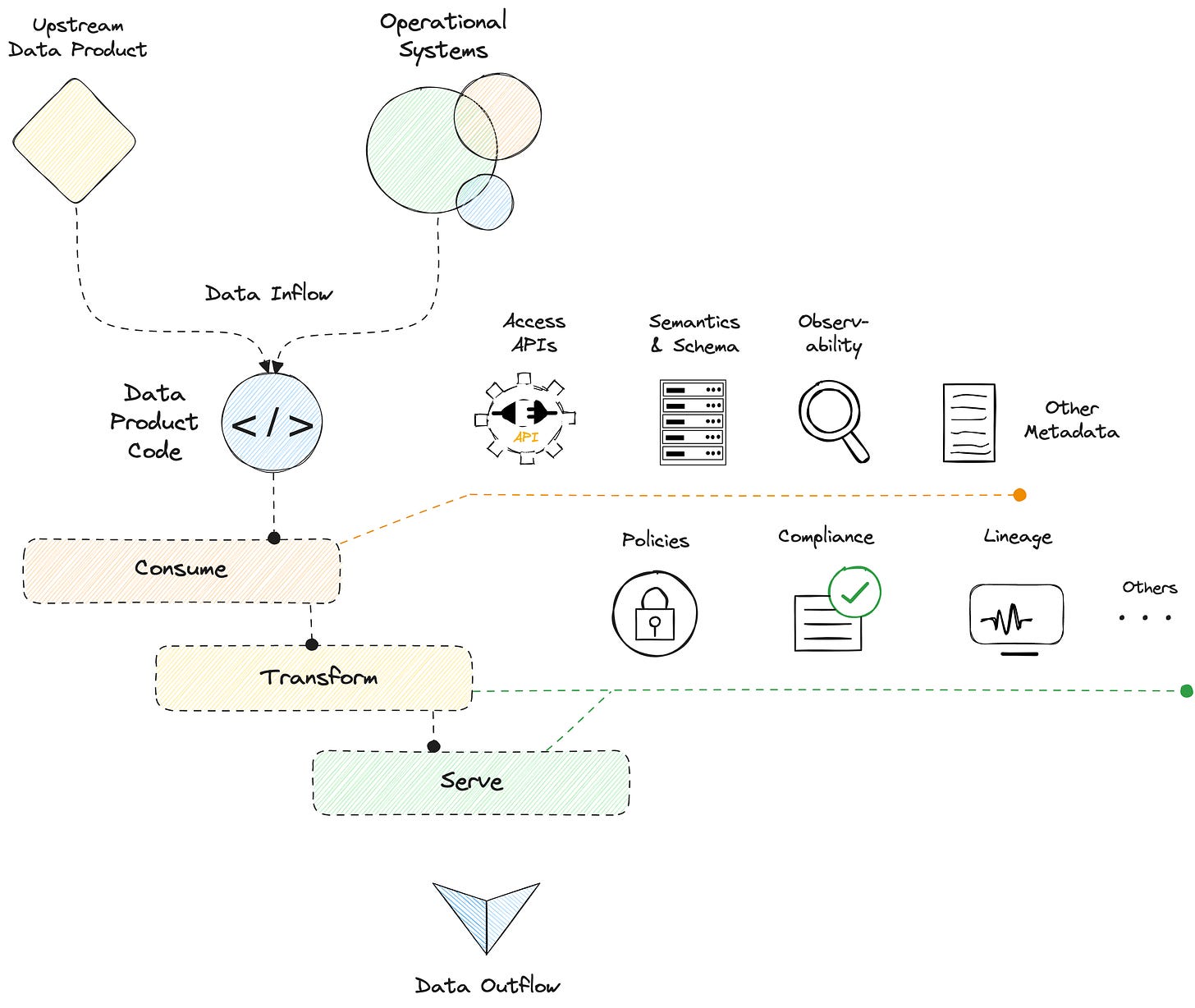
This approach to the data mesh empowers organizations to scale their data capabilities more effectively, improve data quality, and create more value from their data. More about data products as an architectural quantum here.
Second, data products are atomic, functionally cohesive units. Each data product should have its own development lifecycle, including design, deployment, testing and maintenance. This way, data products can be easily combined and reused in new ways to create more sophisticated insights or support new use cases.
With that in mind, let’s explore how data engineering teams can identify, build, and prioritize data products while creating effective boundaries between them.
Before diving in, a note for the ones new here. To exchange ideas and become part of these conversations, join our community channels on LinkedIn & Twitter. You’ll also get immediate access to weekly updates on the data industry, trends, jobs, innovations, and more! 💬
Lean Value Tree
Lean Value Tree (LVT) is a tool for defining outcome-based strategies. In the context of data products, teams can use the LVT to map their development objectives to business priorities, establishing a clear value proposition and domain-driven product boundaries.
They can also leverage LVTs to prioritize use cases for data products instead of being swayed by the underlying data sets. Then, for each of these use cases, they can outline the requirements for the data product, such as the necessary features and data points.
For more on using the lean value tree to build data products, consider the Data Mesh Accelerator Workshop. A practical case study of how Roche used the data mesh framework is available here.
Data Product Interaction Map
By grouping together related use cases, teams can identify natural clusters of data needs. These can help them define the boundaries of a data product. We can use these clusters to create data product interaction maps to see how data sources and integration sources feed into source-oriented and consumer-oriented data products.
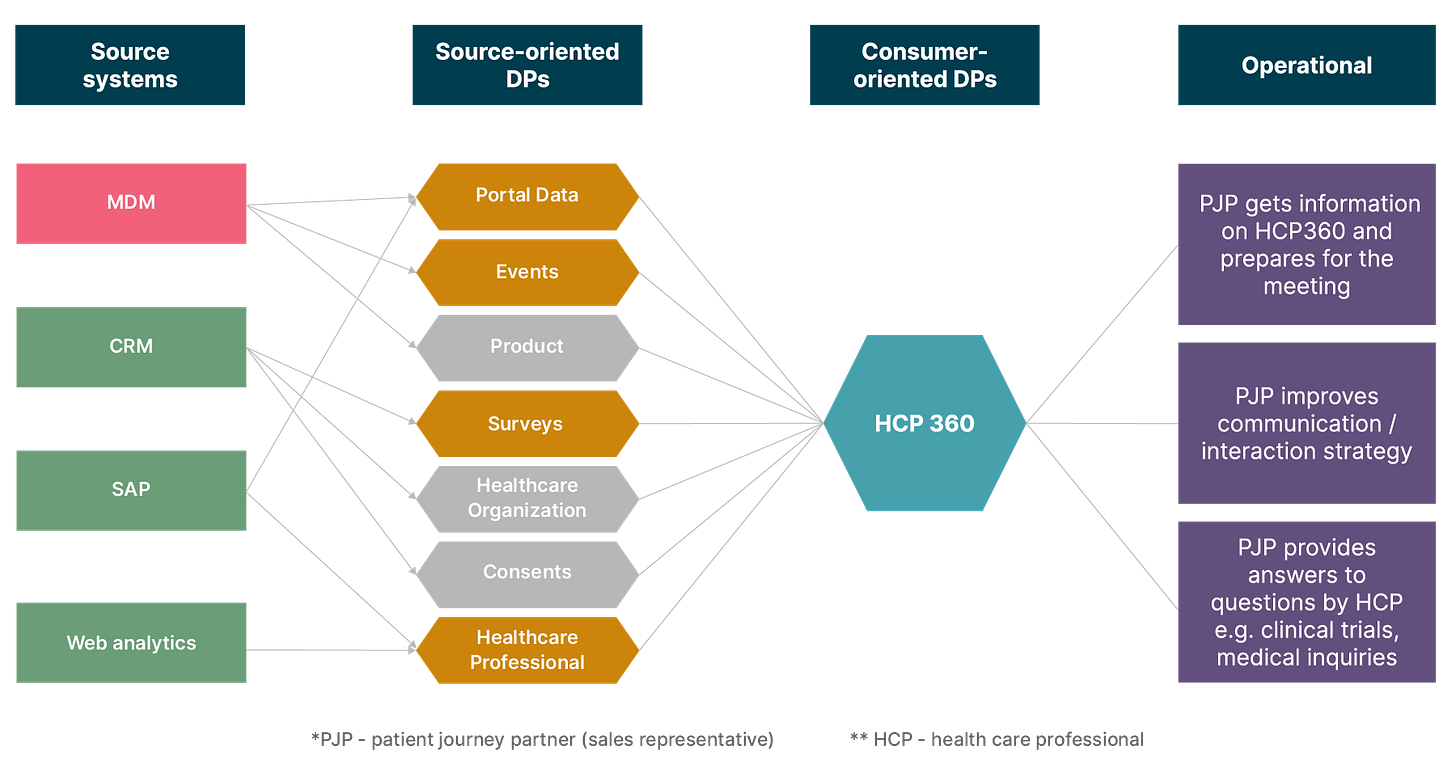
LVT and data product interaction maps help us discover and analyze clusters of data usage requirements across different consumer personas. It also helps us identify seemingly similar data sets that may exhibit varying usage patterns and data access requirements.
For instance, my employer ThoughtWorks as an organization generates data related to new signed deals and upcoming delivery demands using a SAP application (I don’t know what they actually use). This data is valuable for both staffing and hiring folks as well as the Finance team to provide quarterly or annual forecasts about our business.
With this example it’s clearly evident that different attributes of the same data can be consumed with different timeline, completeness and other quality metric expectations by different teams. This introduces our second tool.
Data SLAs for Data Product Atomicity
Within any organization, the needs and expectations of various groups of consumers around data availability and timeliness (among other issues). will vary. This variance highlights the importance of Service Level Agreements (SLAs) in defining the boundaries of data products.
Data SLAs lay down explicit consumer expectations across metrics for data availability, volume, freshness, completeness, and accuracy. In a data mesh, SLAs help identify the appropriate split of data products within a cluster, ensuring they meet the needs of different data consumers.
This way, SLAs build trust between data product providers and consumers, forming the foundation for effective collaboration and utilization of data products in the organization.
Let’s take the simplified example of an e-commerce platform to illustrate how this works in practice. In an e-commerce platform, sales data is used by different teams for different use cases, such as the following:
Dynamic pricing team for pricing strategy. They need real-time updates, which means their focus is timeliness.
Order fulfillment team for warehousing and delivery optimizations. They need all the data available, including failed and canceled orders. Their focus is completeness.
Subsequently, the SLAs for these data products need to be different. Splitting the sales data into multiple data products, each designed to meet the specific SLA requirements of different use cases helps define product boundaries.
It ensures that each team receives the data they need in the desired format and within the expected timeframe, leading to improved operational efficiency and better decision-making across the organization.
It is also important to recognize that the needs and possibilities of data consumption and the requirements of SLAs/SLOs (Service Level Agreements/Service Level Objectives) may not remain static; as time progresses, the optimal data product may also need to adapt and evolve. For instance, as the volume of data processed by a data product increases, the SLOs for that product might need adjustments.
As new use cases present themselves or the existing ones evolve, additional data points may need to be incorporated into the data product to accommodate these new demands. This is why it’s important to continuously monitor and re-evaluate the design and implementation of data products to ensure they continue to deliver value and meet business needs over time. You can read more about SLAs in data product thinking here.
Note from Editor📝: Related read
In essence, the data mesh model recommends teams create multiple data products — atomic, independent and use case-driven — to leverage the most value from data. This delivers several benefits.
Increased efficiency: Creating multiple data products for the same data source enables targeted and efficient data delivery, reducing waste in providing or processing data for consumers.
Improved data quality: Multiple data products enable better control and governance, making it easier to maintain high data quality with independent data validation, cleansing and quality assurance processes.
Simplicity and ease of use: Having multiple data products keeps the data landscape simple and avoids over-complication or over-engineering. This simplicity promotes a better user experience and reduces the cognitive load on data product teams and consumers.
Horizontal scalability and organic evolution: New data products can be added or existing ones modified without affecting the entire system. This flexibility allows the data architecture to evolve organically.
However, it’s extremely common for blurry boundaries between data products to persist if organizations either define them too broadly or narrowly. On the one hand, defining data product boundaries broadly affects their ability to be managed and modified independently, failing the very objective of the data mesh architecture. On the other hand, defining them too narrowly will lead to over-granularity, excessive fragmentation of data products, complexity, and inefficiency.
Having effective product atomicity is critical to successful data mesh implementation. Fortunately, there are a number of tools that can help ensure data products do not blur into one another. Lean value trees, product interaction maps, and SLAs, in particular, can help teams set data product boundaries effectively.
Special call out to my colleagues Ammara, Ecem, and Manisha for tech reviewing my thoughts.
Note from Editor📝: More reads from Manisha on MD101 ➡️
Author Connect
We highly appreciate this contribution from by Ayush Sharma! Feel free to connect with him and learn more about Data Products and Data Mesh 💠
Find me on LinkedIn and Github.