Data Product Mart? Can Data Really be treated as a Product | Issue #15
Short Answer: Yes. Read on for the long version
Hi, welcome to Modern Data 101! If you’re new here, this is an all-in-one community newsletter to enhance your data IQ. We curate insights, ideas, and innovations to help data practitioners and leaders squeeze every drop of value from their organization's data, all while fostering a global community of data trailblazers. But in essence, today modern data comes down to Data Products - product thinking and software principles applied to the realm of data; and we’re here to talk all about it.
Editorial 🤓
The Data Product has become a fiery topic in the community, and given its novelty, several interpretations keep popping up. I’ve even encountered experienced data experts, practitioners, product teams, and executives who struggle with this idea due to a lack of relatability. So, let’s bring down this whole act to something we can all relate to - A retail mart.
We are extremely well-versed in purchasing ‘products’, even digital ones. When we enter a mart, we are aware of what we need, what we want, and run every product through a checklist at the back of our minds. In essence, we are walking data scanners, approving quality on the go to optimise our consumption.
Let’s say you’re buying cereals. Would you pick up a handful of cereals strewn on a rack or a box of cereals that shows when it was manufactured, how to consume it, what ingredients it has, when it expires, and more? There’s a reason it is in a box. When we approach data today, it is like a handful of cereals strewn on the floor. Instead, we are looking for data in a container that gives us all the context around it that makes it fit for consumption.
Here’s a very wild take on what a data product mart would look like. And thanks to some of our colleagues at ThoughtWorks, we were able to see how effective a mart-y design could be for data consumption.
Definitely zoom into this picture for better clarity. In essence, this directional illustration talks about productizing data.
Makes data discoverable through appropriate titles, descriptions, tags, filters, and categories.
Makes data addressable across the data ecosystem through unique data links
Makes data understandable through rich semantic data - descriptions, tags, data profiles, usage metrics, and more
Makes data natively accessible through output ports with connectivity to the user’s specific domain. Users can direct connect and consume data by selecting the required port.
Makes data trustworthy through approval marks, quality scores, importance scores, ranks, ratings, filters, and more.
Makes data interoperable by giving data products and data within the data products to communicate with each other and external entities.
Makes data independently consumable with complete self-service and no dependencies on external disruptive agents. Every dependency is contained within the realm of the data product.
Makes data secure by applying the necessary access and masking policies.
The mart design does not necessarily enable all these qualities except, say, discoverability and accessibility. These capabilities are enabled by the underlying infrastructure and code that are subsets of the data product and not visible on the high-level consumption-oriented design.
If you’re curious about the infra and code subsets of a data product, refer to datadeveloperplatform.org for the community-driven Data Developer Platform specification. While DDP is not the only way to enable Data Products, it is a seamless non-disruptive infra piece to get the Data Product Ecosystem up and running.
You can’t necessarily talk about Data Products without talking about the code and infrastructure aspects, so stay tuned for when we dive deeper into these segments!
Community Space 🫂
We’ve always had a lot of inspiration from the community and often source resonating ideas from the larger group. So it was high time to create a dedicated space for all the voices that have been shifting the needle and can help us go a step further in our data journey.
A couple of months ago,
talked about the blueprint for creating data products. The approach has been neatly dissected into two steps:Leveraging Product Management Mindset in Data Creation
Applying Product Management to Data Presentation and Delivery
Adopting a product management mindset prior to data creation marks the initial stage towards providing dependable data products. However, that alone is NOT sufficient. In real life, once a product is created, it then needs to be packaged and presented to customers.
Packaging the data and making it fit for long-term consumption sits top of the ToDos!
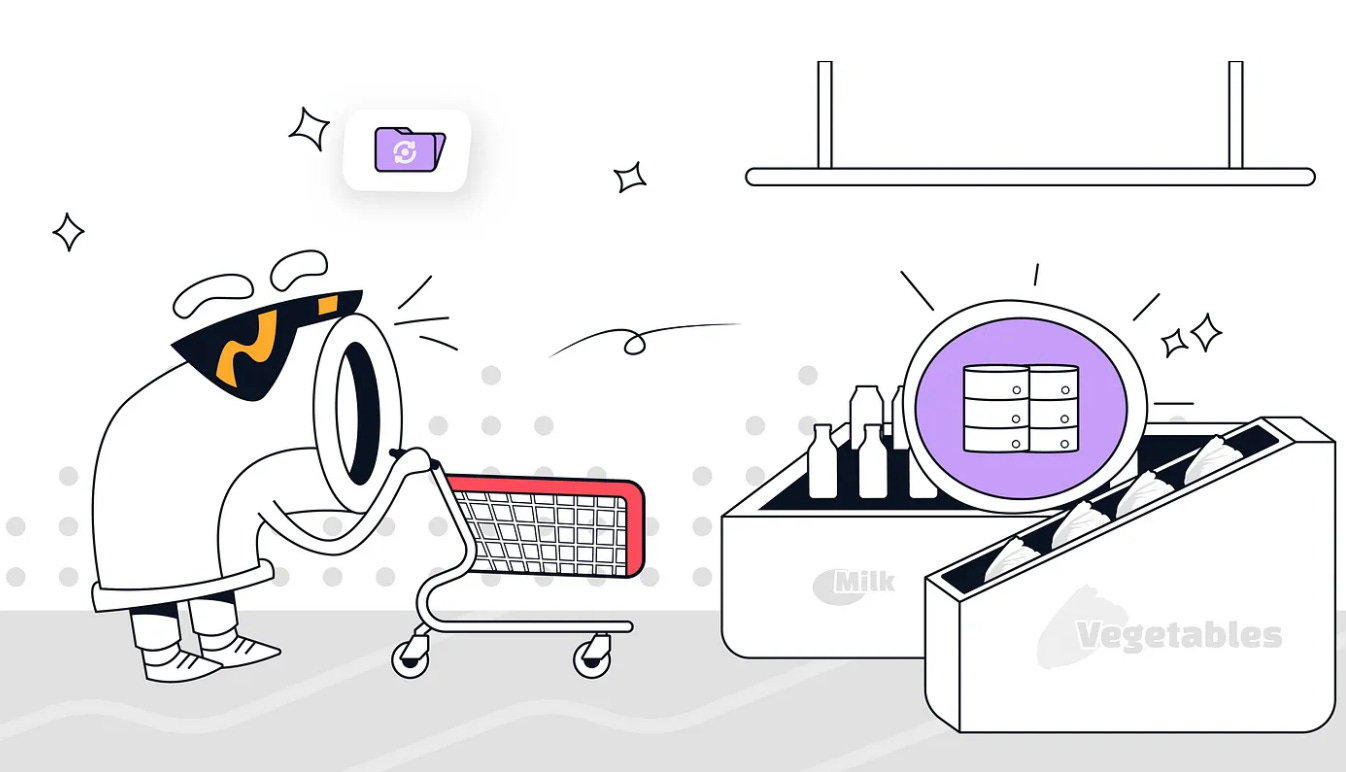
The later half of the piece breaks down each of the qualities we talked about in the mart scenario- discoverability to security.
Jeffrey T. Pollock went a step further not just to define data as a product, but data as capital - in the analogy that you buy the product and put it back into, say, another product to create business value.
So, for old-timers like me, why does Data Product Thinking seem fresh and cool? We’ve spent the past 20+ years promoting Data Governance, Infonomics and Value of Data… we get it, right?
Well, yes and no. I for one really like this new turn of phrase – Data Product Thinking – precisely because it changes the point of emphasis around data value. Here’s what I mean:
An “asset” is something to be governed and managed
A “capital asset” is something to be counted and accounted for
With this analogy of data as capital on top of data products, Jeffrey emphasises how the product is meant to achieve a task. “The ‘product bias’ is towards action – the act of creating something that does a job.”
This piece also focuses on the packaging of data for consumption: “It must exist within a lifecycle that has the familiar attributes of physical products in the commercial realm.”
Announcements 📢
A lot has been cooking this month, but first, our most awaited announcement of the year- Modern has entered into a strategic partnership with ThoughtWorks!
If you’ve followed our work for some time, you've probably noticed how I’ve always admired the commendable approach of ThoughtWorks to all things engineering. Cue all those countless excerpts from Martin Fowler and TW! This partnership is, therefore, not just “strategic” per se but also personal and very close to our way of work and thinking.
ThoughtWorks has been dabbling with 𝐃𝐚𝐭𝐚 𝐏𝐫𝐨𝐝𝐮𝐜𝐭𝐬 𝐚𝐧𝐝 𝐃𝐚𝐭𝐚 𝐌𝐞𝐬𝐡 long before it was popular and has been deploying these frameworks for their customers with commendable results ever since.
The combination of Thoughtworks' world-class data engineering and AI practices and Modern's paradigm-changing DataOS Platform, designed to streamline the creation and management of Data Products, is poised to reshape the future of data.
You can read all about this partnership here!
Secondly, we ventured into presenting a joint session with ThoughtWorks at CDOIQ’s 17th Annual Symposium in Boston this year, and it turned out to be more than just data with tons of good times and good food!
Thanks for Reading 💌
Here’s a breather for you for sticking around till the end!
Follow for more on LinkedIn and Twitter to get the latest updates on what's buzzing in the modern data space.
Feel free to reach out to us on this email or reply with your feedback/queries regarding modern data landscapes. Don’t hesitate to share your much-valued input!
ModernData101 has garnered a select group of Data Leaders and Practitioners among its readership. We’d love to welcome more experts in the field to share their stories here and connect with more folks building for the better. If you have a story to tell, feel free to email us!